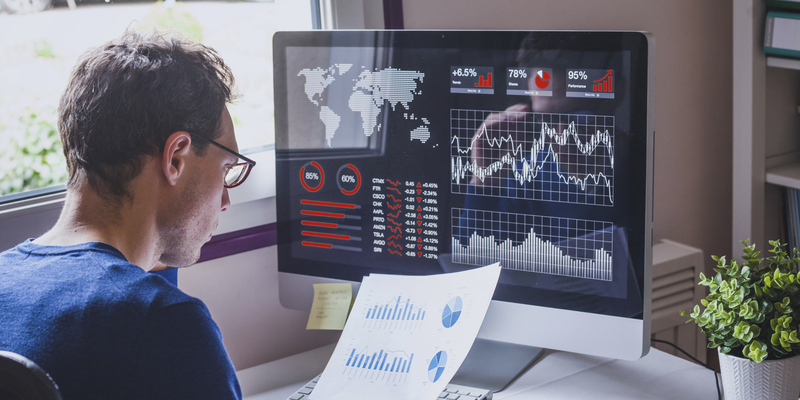
Dirty data is a common issue for organizations using analytics to address business and workforce challenges. Data cleansing can scrub dirty data clean, helping ensure more accurate, more complete insights and maintaining confidence in the analytics process overall.
Access to reliable data is predicted to top business and HR priority lists in 2022 as more enterprises start using people analytics to address organizational challenges. But how do organizations ensure their new and existing data is reliable and therefore ready for analysis? Enter: data cleansing. Proactively and reactively cleaning that data is a start. You see, there's a filthy threat to reliable data-driven insights lurking in the columns and rows of analytics databases everywhere. That mucky menace is known as dirty data.
Data tends to become incorrect, inaccurate, incomplete, inconsistent or duplicative — dirty — over time. Analyses of this data can lead to misunderstandings about an organization's people and processes, engendering poorly informed decisions that negatively impact those people and processes and undermining trust in analytics processes in general. If organizations know that their data is dirty, they can harness the power of data cleansing to pressure wash the mold and grime off their unclean datasets, priming them for more reliable analyses.
What is dirty data?
Dirty data refers to incorrect, inaccurate, incomplete, inconsistent or duplicative data. Data becomes dirty in three main ways: user error, poor interdepartmental communication and inadequate data strategy. If not cleaned, dirty data may lead to incorrect beliefs and assumptions about data-driven insights, poorly informed decisions based on those insights and distrust in the analytics process overall. It can also adversely impact operations reliant on clean data to execute correctly.
One example of dirty data is incorrect employee mailing addresses. Suppose that 121 Waverly Lane was entered as an employee's mailing address, even though 123 Waverly Lane is the employee's correct mailing address. This employee's address data is incorrect, so it's considered dirty. An example of an unfavorable outcome from using this dirty data would be an organization consistently mailing the employee's correspondence to the wrong address, which could frustrate both the employee and the employer.
What is data cleansing?
Data cleansing, also known as data cleaning, is the process of cleaning dirty data by resolving instances of incorrect, inaccurate, incomplete, inconsistent or duplicative data. An example of data cleansing is an organization that wants to understand the gender representation of its workforce, but its employee dataset has missing gender values for many employees. Unfortunately, analyses of the gender representation of this workforce would be incomplete until the missing values were filled in. This completion of blank values is just one of many aspects of data cleansing.
Here's another example of data cleansing: Suppose a company's employee database contains many different classifications for employees with high school diplomas. Employee education levels are tagged as "high school diploma," "high school," "HIGH SCHOOL" and "secondary school." Now suppose someone pulls this data for a short-notice meeting about the education-level predominance of the company's workforce. Unfortunately, only one of the classifications was selected, resulting in an inaccurate percentage of employees with high school diplomas. Data cleansing can help prevent similar cases of dirty-data analysis by:
- Removing classification variations altogether, leaving only, for example, "high school diploma"
- Grouping similar classifications and other potential variations into a single "high school diploma" group or family
What is the purpose of data cleansing?
The purpose of data cleansing is to improve data quality by resolving instances of dirty data. Dirty data can be a damaging data quality issue for any business, especially those using analyzed data to make decisions about people and everyday processes and operations. Dirty data is also an expensive problem, costing businesses between 15 and 25 percent of revenue. And because analyses of dirty data can lead to poorly informed decisions that negatively impact employees and business processes, leaders should address any instances of dirty data sooner rather than later.
"If your data's not complete or accurate, there are bad decisions being made and misrepresentations of facts," says Kristin Hlavinka, director of content and data governance at ADP. "Not only are you making bad decisions, but you could be presenting statistics higher up that are inaccurate."
Large amounts of dirty data likely mean that an organization's data cleansing practices need attention. Committing to proactively and reactively correcting data inaccuracies, filling in missing values and removing or grouping inconsistent values is a start to cleaner data and more accurate, more complete insights. For the most efficient results, business leaders should look for innovative data quality automation capabilities that make cleansing dirty data as simple as notifying analysts when data is dirty and what steps to take to cleanse it.
Why is data cleansing important?
Data cleansing is important because dirty data can cause misunderstandings about an organization's people and processes, so cleansing it is essential. Discovery and cleansing of dirty data are also critical to analytics professionals who may not know their insights are inaccurate or are trying to understand why. Dirty data can even cause inaccurate insights to be blamed on both the analytics technology crunching the data and the data that's being crunched, says Brent Weiss, senior director of product management.
"When a user starts to say, 'There's something wrong with this data,' they're now going to start to say, 'I can't trust the analytics.' So, everything else just falls apart," Weiss says. "It doesn't matter how big the chart is or how it compares to something else because, at the end of the day, you're like, 'I don't know if this is accurate.' That perception of accuracy is paramount. There are multiple ways that can lead a person not to trust the data."
How do you perform data cleansing?
You perform data cleansing by resolving instances of incorrect, inaccurate, incomplete, inconsistent or duplicative data. The journey to consistently clean, high-quality data starts with your data entry system, Hlavinka says.
"There are two approaches to data cleansing: getting the data right from the beginning, which alleviates a lot of back-end pain, or you clean the data before you profile, analyze and add value to it," Hlavinka says. "It's an iterative process of finding problems, understanding the root cause of those problems, fixing it for the short-term and then fixing it for the long-term so that it doesn't happen again."
Other proactive and reactive steps leaders can take to help ensure their data gets cleaned, include:
- Looking beyond the percentages. Go under the hood and look at the details in the data to see if it contains spelling or punctuation errors, incorrect formatting or duplicates
- Pressure-testing accuracy before presentations by sharing data and associated insights with others. They may notice something you didn't.
- Addressing blank values in a dataset. "That's the least complex," Weiss says. "If something's missing, that's fundamentally going to undermine the quality of your analytics."
- Identifying and questioning any logical inconsistencies in datasets or analyses. For example, suppose you have an employee headcount of one for an entire department. Does this headcount make sense for that department? If not, could this be a data entry issue, an issue with a rule in the dataset or some other issue?
- Employing data quality experts who regularly audit data and manage data cleaning, validation and governance
Preventing garbage in, garbage out with data cleansing
Unclean data analyses come out if unclean data goes in. If data is dirty and no one knows about it or cleans it, business and HR leaders risk making faulty decisions based on inaccurate data. Meanwhile, the analytics professionals presenting this data may have to grapple with distrust from leaders who recognize that something about their data isn't quite right.
Automated technology that analyzes databases for dirty data can help ensure that analysts provide the most high-quality insights to leaders who rely on data to make decisions. Meanwhile, data quality indicators are already built into ADP's Diversity Dashboard.
This story originally published on SPARK, a blog designed for you and your people by ADP®.